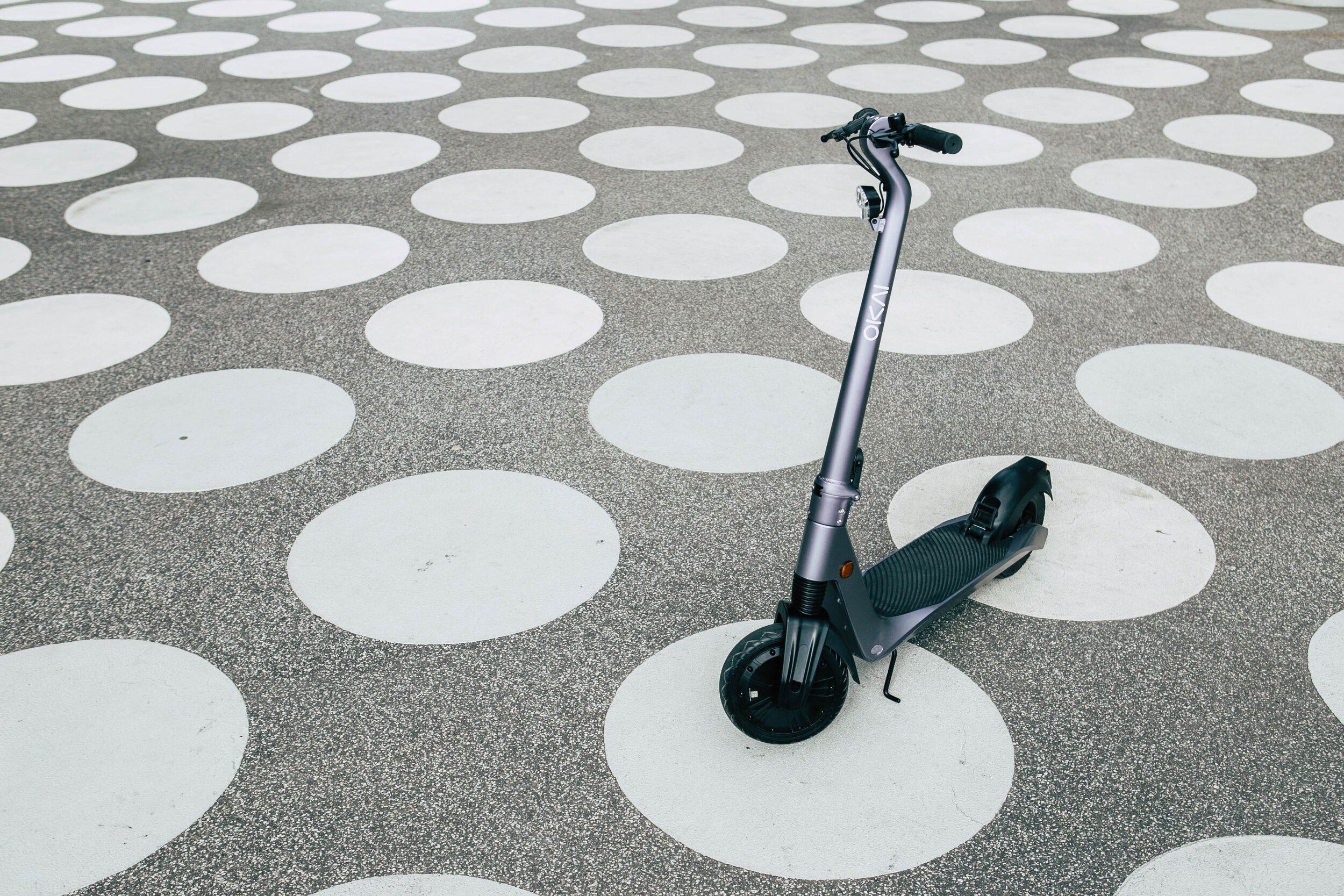
Preventing Vandalism and Theft in the Sharing Economy
Overview
According to the Boston Consulting Group (BCG), electric scooters have an average life-span of only three months. This is mainly due to acts of misuse, vandalism, and theft. These issues directly affect the financial performance and brand reputation of e-scooters businesses, raising environmental concerns.
By analyzing historical data, it’s possible to identify patterns that help companies understand the risk of a particular e-scooter being stolen. These patterns are essential to perform data-driven decisions in business. A simple solution would be to use traditional statistics, such as the number of incidents by location, to identify the areas of high risk. But what if we can associate temporal data with geographic data?
Challenge
This internationally known company in the electric scooter rental business was constantly confronted with issues such as thefts and acts of vandalism that made the business unfeasible. They even tried to resort to less personalized solutions, but without success.
Due to the nature of the crime prediction problem, the use of simple statistics can be more of an obstacle than a benefit. Simple statistics do not take into account the dynamism and relativity of the continuous change in crime factors.
Lisbon,
Portugal
Opportunity
EAI's specialists created several risk scoring algorithms that help companies identify and prevent crime events and misuse of the systems. The core of these systems is a classification model that can predict the risk of each electronic scooter with much greater success.
There are several reasons for using data science to solve this problem. Some of the main advantages of this project were the perfect separation of geographical areas, the perfect division of time, the use of weak elements, and a risk scoring output. At the end of the project, it was also possible to identify the compartment patterns of each region according to the time.