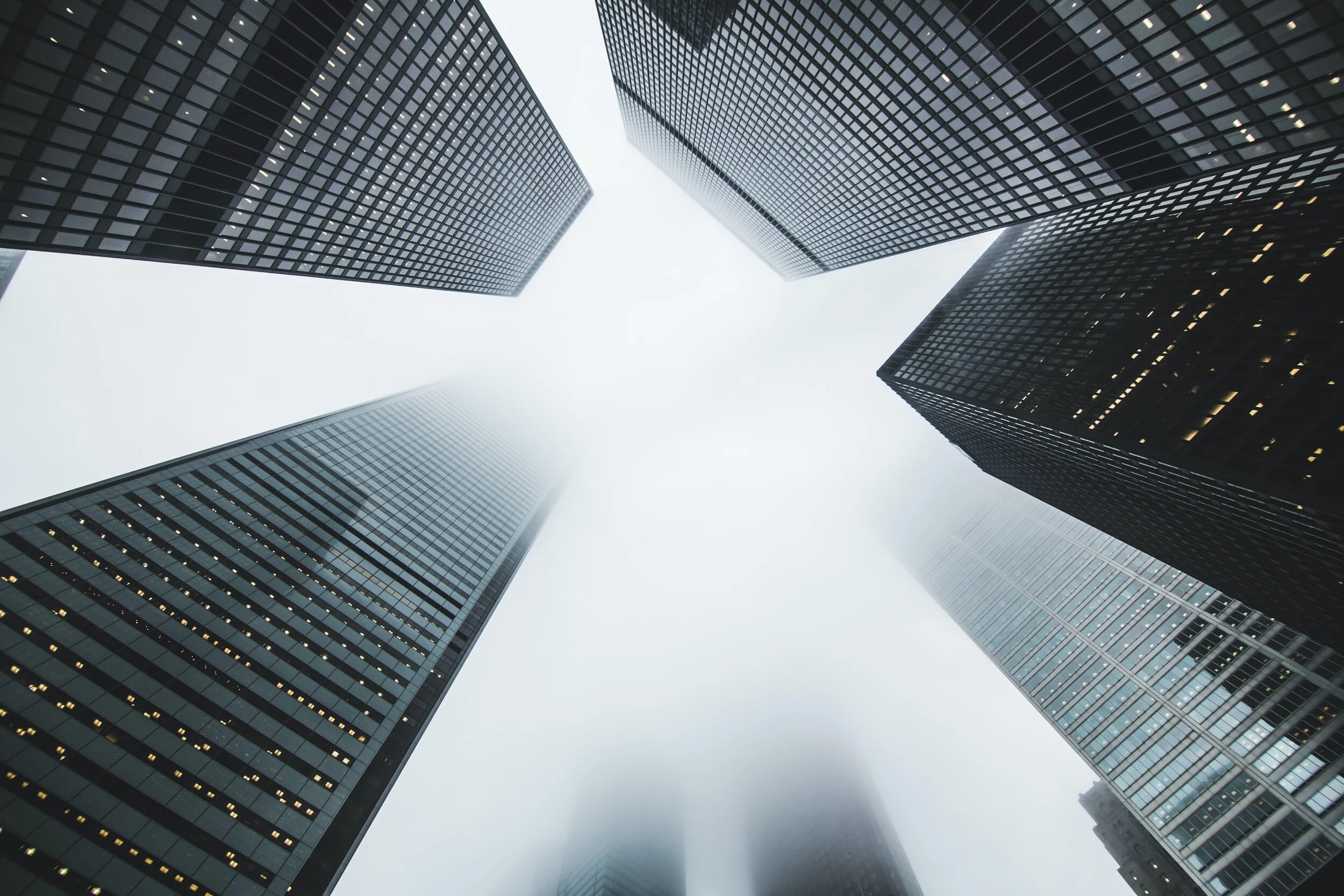
Data-driven Decision Making
What is Data-Driven Decision Making
Data-driven decision-making (DDD) is a process that involves using data and analytics to inform and support business decisions. By relying on data and analytics to provide objective, evidence-based insights, businesses can make more informed, strategic decisions, and optimize their operations and processes to improve their performance and outcomes. DDD is an important tool for businesses in an increasingly data-driven world and can help organizations stay competitive and successful in an ever-changing market.
Data-driven Decision Making (DDD) is the practice of basing decisions on the analysis of data, rather than intuition. It’s like piloting a plane by looking at the instruments, instead of looking out the window.
Example: a marketer could select the products to promote purely based on their experience in the field, however, with some supporting data coming from sales or website analytics, that same marketer would be able to recognize patterns and make better-informed decisions, increasing the effectiveness of the product promotions.
This article is separated into the following chapters:
Benefits of data-driven decision making
Examples of data-driven decision making
Challenges and pitfalls of data-driven decision making
Best practices for implementing data-driven decision making
Conclusion
Benefits of data-driven decision making
Data-driven decision making (DDD) has various advantages and can have a substantial influence on a company's performance and outcomes.
The benefits of data-driven decision-making have been demonstrated conclusively:
Improved decision making: According to an Accenture report, firms that use data-driven decision making are roughly six times more likely to make faster choices and four times more likely to make better judgments than organizations that do not use DDD.
Increased efficiency: According to a McKinsey study, data-driven firms are 23 times more likely to attract consumers and 19 times more likely to be profitable than non-DDD organizations.
Enhanced customer satisfaction: According to a Deloitte report, data-driven firms are three times more likely to exceed customer expectations and twice as likely to retain customers than non-DDD organizations.
Greater sales: According to a Forbes survey, companies that use data-driven decision making have higher revenues and valuations than those that do not use DDD.
High productivity: A study from MIT and Penn’s Wharton School shows that statistically, the more data-driven a firm is, the more productive it is—even taking into account a possible range of confounding factors. There was a high correlation between return on assets, return on equity, asset utilization, and market value. The relationship seems to be causal, that is, being data-driven causes the improvements, not the other way around. They found companies that adopt Data-driven Decision Making have a 5 to 6% higher productivity than what would be expected given their other investments and information technology usage.
Overall, the advantages of data-driven decision-making are substantial and may have a considerable influence on a company's performance and outcomes.
Businesses can make better, quicker choices, boost efficiency and profitability, and improve customer happiness and retention by leveraging data and analytics to inform and assist their decision-making.
Examples of data-driven decision making
The following are some examples of data-driven decision-making in various businesses and contexts:
Retail: To detect trends and patterns in client preferences and purchasing behaviors, a retail organization may employ data-driven decision-making to study customer purchase history and activity. This data might be used to offer more focused, tailored suggestions to consumers, or it could be used to modify the company's product mix and price to better correspond with customer demand.
Finance: A financial services firm may employ data-driven decision-making to assess consumer creditworthiness and risk in order to make better educated, objective lending and credit choices. This data might be utilized to detect possible credit concerns, establish suitable credit limits and conditions, and create more effective risk management techniques.
Healthcare: In order to detect trends and patterns in patient health and treatment, a healthcare institution may employ data-driven decision-making to examine patient medical records and data. This data might be used to improve the accuracy and efficacy of medical diagnoses, highlight possible hazards or problems, and provide more customized effective treatment programs.
Manufacturing: manufacturing companies may utilize data-driven decision-making to evaluate production data in order to discover inefficiencies and bottlenecks in the manufacturing process. This data might be utilized to optimize the manufacturing process, eliminate waste and enhance efficiency, and find cost-cutting and efficiency-improvement possibilities.
Overall, data-driven decision-making may be applied in a wide range of sectors and situations, providing useful insights and perspectives that can assist firms in making better, more informed decisions.
Challenges and pitfalls of data-driven decision making
While data-driven decision-making (DDD) has numerous advantages, it also has several drawbacks that businesses should be aware of while using DDD. DDD's significant problems and drawbacks include the following:
Lack of high-quality data: For data-driven decision-making to be effective, companies must have access to high-quality data that is correct, relevant, and complete. However, many companies fail to obtain and keep high-quality data, making DDD application problematic.
Biases and overfitting: Biases and overfitting can occur in data-driven decision-making, leading to inaccurate or misleading findings. For example, if an organization's data is not representative of the larger community, or if the data is not adequately processed, the conclusions obtained may be prejudiced or inaccurate.
Difficulty implementing: Data-drive decision-making can be difficult to adopt since it needs businesses to have strong data literacy and analytics capabilities, as well as excellent data governance and management. Organizations that lack these characteristics may struggle to implement DDD successfully or may not be able to fully enjoy the benefits of DDD.
Overall, while data-driven decision-making has numerous advantages, companies must be aware of the possible problems and pitfalls of DDD and take actions to solve these challenges in order to effectively employ DDD.
Best practices for implementing data-driven decision making
Here are some best practices for implementing data-driven decision-making (DDD) in an organization:
Develop a clear understanding of the goals and objectives of DDD: Before implementing DDD, companies must have a clear understanding of DDD's aims and objectives, as well as how they will assist the organization to accomplish its larger business objectives. This may assist ensure that DDD is aligned with the overall strategy and direction of the business, as well as that DDD is deployed in a way that provides value and benefits to the organization.
Invest in data literacy and analytics skills: In order to properly gather, analyze, and understand data, companies must have excellent data literacy and analytics capabilities. Organizations should invest in training and development programs to assist people in developing these abilities, and they should think about hiring a team of data scientists and other analytics specialists to support DDD projects.
Establish effective data governance and management: Organizations must have robust data governance and management policies in place for DDD to be effective. This includes creating defined roles and responsibilities for data stewardship and management, as well as setting policies and processes for managing and preserving data.
Foster collaboration and partnerships: Collaboration and relationships with other businesses, such as data suppliers, analytics vendors, and technology partners, are frequently required while implementing DDD. Organizations should create strong connections with these partners and clear, effective procedures for working and sharing data and analytics resources.
Implementing data-driven decision-making can be challenging, but by following these best practices, organizations can ensure that DDD is implemented effectively and provides value to the organization.
Conclusion
In conclusion, data-driven decision-making (DDD) is a valuable approach for businesses that want to use data and analytics to inform and support their decision-making. Businesses may make better informed, strategic choices and optimize their operations and processes to enhance their performance and outcomes by relying on data and analytics to deliver objective, evidence-based insights.
However, implementing DDD may be difficult, and companies must be aware of the possible problems and pitfalls of DDD, such as the requirement for high-quality data, the risk of bias and overfitting, and the importance of appropriate data governance and management. Organizations can use DDD effectively and reap the full benefits of this approach to decision-making by following best practices for implementing DDD, such as developing a clear understanding of the goals and objectives of DDD, investing in data literacy and analytics skills, and fostering collaboration and partnerships.
If you're interested in implementing data-driven decision-making in your organization and want to learn more about how Enlightenment.ai can help, please don't hesitate to contact us. We would be happy to discuss your needs and how we can support your data-driven decision-making initiatives.